This post is the last in our series of 5 blog posts highlighting use case presentations from the 2nd Edition of Seville Machine Learning School (MLSEV). You may also check out the previous posts about the 6 Challenges of Machine Learning, Predicting Oil Temperature Anomalies in a Tunnel Boring Machine, Optimization of Passenger Waiting Time for Elevators, or Applying Topic Modeling to improve Call Center Operations.
Today, we delve into a use case from the chemicals industry originally presented by José Cárdenas, Technical Services Manager at Indorama Ventures. Headquartered in Bangkok, Thailand, Indorama Ventures started its journey in 1994 specializing in the production of worsted wool yarn, which is typically used in tailored garments and textiles such as suits. Gradually, the company completed its global expansion with acquisitions in the United States and Europe, eventually becoming a global PET (Polyethylene Terephthalate) producer as well as a sizable player in the PTA (Purified Terephthalic Acid) business. Today, Indorama operates production sites in 31 countries on five continents – in Africa, Americas, Asia, Europe & Eurasia.
PET is manufactured in the form of pellets that are then melted to produce packaging material (food and beverage containers, bottles) and various polyester fibers consumed in industries from automotive to medical. The specific Machine Learning project Indorama tackled involved the carboxylic acid process, which has a critical role in PET production of various grades, e.g., hot fill, high/low intrinsic viscosity, quick heat, general grade.

In this project, the Indorama team was mainly concerned with the optimization of the above process, which can be translated as achieving cost reductions while not compromising from key PET quality parameters. In other words, it’s the age-old “do more with less” challenge, whereby the inputs to the process are used more efficiently than it is in the status quo.
Fortunately, the project team had access to 2.5 years worth of detailed chemical process data containing more than 6 million data points. This highly technical dataset described many aspects of the complex chemical process such as throughput, catalyst material concentration, feed to air ratio, oxygen level, and more. In order to pick apart the best signals out of it, technical domain experts turned to anomaly detection, outlier elimination, and association discovery by applying BigML’s handy unsupervised methods. Understanding the variable correlations during the data exploration phase was also key in feature selection to further eliminate noise.
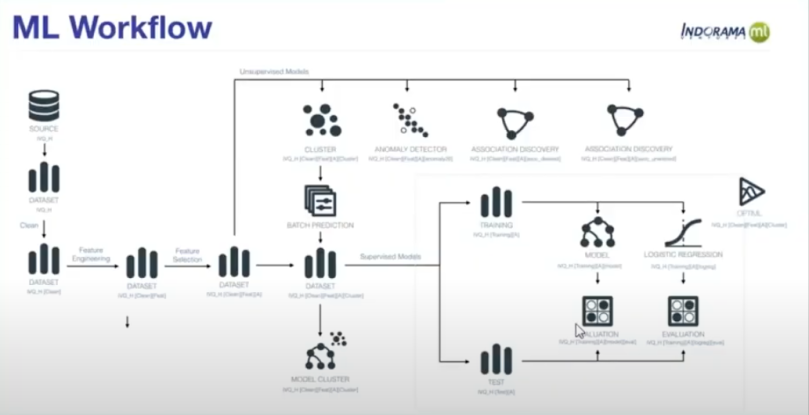
The Indorama team went through multiple iterations to improve their classification model metrics such as recall to acceptable levels. The team of experts used BigML’s Partial Dependence Plot (PDP) visualizations to analyze the fine-grained impact of combinations of process variables on the PET quality and yield. In return for all the hard work, such close-up model inspection resulted in discoveries even long-time chemical process experts were not previously familiar with. These days they are hard at work in making the necessary changes and upgrades to the underlying chemical processes to mimic the higher efficiency modes of operation predicted by their best performing BigML models some of which were built by OptiML — BigML’s popular ‘AutoML’ capability.
With that, let’s jump into the corresponding MLSEV video describing exactly how Indorama went about implementing their custom Machine Learning workflow and how the subsequent iterations helped them gain actionable insights:
Do you have a similar Industrial Process Optimization challenge?
Depending on your specific needs, BigML provides expert help and consultation services in a wide range of formats including Customized Assistance to turn-key smart application delivery — all built on BigML’s market-leading Machine Learning platform. Do not hesitate to reach out to us anytime to discuss your specific use case at info@bigml.com.